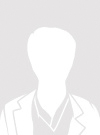
Contributions
Abstract: EP911
Type: E-Poster Presentation
Session title: Myelodysplastic syndromes - Clinical
Background
Myelodysplastic syndrome (MDS) is an underdiagnosed preleukemic condition mainly affecting patients over the age of sixty. In approximately one-third of patients, MDS evolves into acute myeloid leukemia (AML), which is highly aggressive and can be fatal. Accurate and early MDS diagnosis can allow physicians to monitor patients and provide early treatment, which may delay advancement of MDS and improve quality of life in many patient populations. However, MDS is often unrecognized by primary care physicians and is difficult to distinguish from other causes of bone marrow failures, cytopenias, and other clonal stem cell disorders.
Aims
The purpose of this study is to develop a machine learning algorithm (MLA) for the prediction of MDS one year prior to clinical diagnosis of the disease.
Methods
Retrospective analysis was performed on 790,470 patients over the age of 45 using electronic health records (EHR) drawn from over 700 healthcare sites across the US between 2001 and 2019. Two models, including a gradient boosted decision tree model (XGBoost) and a logistic regression model, were trained to predict onset of MDS using vital signs, lab results and demographics information from up to 2 years of patient EHR data. The models did not require blast percentage and cytogenetics as inputs. Retrospective predictions were made 1 year prior to MDS diagnosis as determined by International Classification of Diseases (ICD) codes. Performance was assessed with regard to area under the receiver operating characteristic curve (AUROC) and a SHAPley analysis of feature importance.
Results
On a hold-out test set, the XGBoost model achieved an AUROC value of 0.87 when tested for prediction of MDS one year prior to MDS diagnosis, at a sensitivity of 0.70, and specificity of 0.88. Age, hematocrit, red blood cell count, and peripheral oxygen saturation were found to be the most important features for MDS prediction. The XGBoost model was compared against a logistic regression model. The logistic regression achieved an AUROC of 0.77, at a sensitivity of 0.75 and specificity of 0.79. Receiver operating characteristic (ROC) curves and comparison of AUROC of the XGBoost (XGB) and logistic regression (LR) models are shown in figure below.
Conclusion
Machine learning methods can be used to accurately predict MDS one year prior to diagnosis using current standard procedures. The algorithm only utilized readily available EHR data, without requiring blast percentages or cytogenetics. In clinical practice, use of such a tool may allow for early diagnosis of MDS and more appropriate treatment administration.
Keyword(s): Diagnosis, MDS, Prediction
Abstract: EP911
Type: E-Poster Presentation
Session title: Myelodysplastic syndromes - Clinical
Background
Myelodysplastic syndrome (MDS) is an underdiagnosed preleukemic condition mainly affecting patients over the age of sixty. In approximately one-third of patients, MDS evolves into acute myeloid leukemia (AML), which is highly aggressive and can be fatal. Accurate and early MDS diagnosis can allow physicians to monitor patients and provide early treatment, which may delay advancement of MDS and improve quality of life in many patient populations. However, MDS is often unrecognized by primary care physicians and is difficult to distinguish from other causes of bone marrow failures, cytopenias, and other clonal stem cell disorders.
Aims
The purpose of this study is to develop a machine learning algorithm (MLA) for the prediction of MDS one year prior to clinical diagnosis of the disease.
Methods
Retrospective analysis was performed on 790,470 patients over the age of 45 using electronic health records (EHR) drawn from over 700 healthcare sites across the US between 2001 and 2019. Two models, including a gradient boosted decision tree model (XGBoost) and a logistic regression model, were trained to predict onset of MDS using vital signs, lab results and demographics information from up to 2 years of patient EHR data. The models did not require blast percentage and cytogenetics as inputs. Retrospective predictions were made 1 year prior to MDS diagnosis as determined by International Classification of Diseases (ICD) codes. Performance was assessed with regard to area under the receiver operating characteristic curve (AUROC) and a SHAPley analysis of feature importance.
Results
On a hold-out test set, the XGBoost model achieved an AUROC value of 0.87 when tested for prediction of MDS one year prior to MDS diagnosis, at a sensitivity of 0.70, and specificity of 0.88. Age, hematocrit, red blood cell count, and peripheral oxygen saturation were found to be the most important features for MDS prediction. The XGBoost model was compared against a logistic regression model. The logistic regression achieved an AUROC of 0.77, at a sensitivity of 0.75 and specificity of 0.79. Receiver operating characteristic (ROC) curves and comparison of AUROC of the XGBoost (XGB) and logistic regression (LR) models are shown in figure below.
Conclusion
Machine learning methods can be used to accurately predict MDS one year prior to diagnosis using current standard procedures. The algorithm only utilized readily available EHR data, without requiring blast percentages or cytogenetics. In clinical practice, use of such a tool may allow for early diagnosis of MDS and more appropriate treatment administration.
Keyword(s): Diagnosis, MDS, Prediction