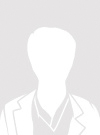
Contributions
Abstract: EP602
Type: E-Poster Presentation
Session title: Chronic lymphocytic leukemia and related disorders - Biology & Translational Research
Background
The international prognostic index for patients with chronic lymphocytic leukemia (CLL-IPI) is the current standard for prognostication. We previously improved the CLL-IPI by 1) using a Treatment and Infection Model (CLL-TIM) and 2) by the number of signaling pathways impaired by recurrent mutations. In this study, we compare the performance of Machine Learning models trained on CLL-IPI variables plus baseline (para)clinical variables with CLL-IPI plus recurrent mutations in patients with newly diagnosed CLL.
Aims
1) Investigating the predictive performance of CLL-IPI variables, recurrent gene mutations, and (para)clinical variables for Treatment at time of CLL diagnosis.
2) Studying the impact of data-driven selection of the Machine Learning algorithm as well as the interaction between data variables and the algorithms.
Methods
In a cohort consisting of 314 patients with newly diagnosed CLL, CLL-IPI variables, recurrent gene mutations, and (para)clinical variables were extracted and used as features in a predictive framework in which a variety of Machine Learning algorithms (Linear SVM, AdaBoost, CatBoost, XGBoost, LightGBM, RandomForest, ExtraTrees, and Bagging) were used to predict CLL treatment as the outcome.
By using different combinations of feature sets, four models were defined as following:
- CLL-IPI features only (IPI),
- CLL-IPI features plus recurrent mutations (MUT),
- CLL-IPI features plus (para)clinical baseline features (BL),
- All the above features (FULL).
Patients were randomly split into a 60% train set and a 40% blinded validation set. The train set was further split 40 times into training set (75%) and test set (25%) to perform hypothesis testing, whereas the validation is still blinded. We here report the preliminary results on the train set.
Results
The preliminary results showed that adding either data on recurrent mutations (MUT) or (para)clinical baseline data (BL) improved the predictive performance significantly compared to the CLL-IPI only (Fig. 1A and 1B). Comparing the BL and MUT models, showed that adding baseline (para)clinical features (BL) predicted CLL treatment better than adding recurrent mutations (MUT) (Fig. 1C). As expected, including all the three feature sets (FULL) outperformed the BL model. For all four hypotheses, the Bagging algorithm achieved the best performance (Fig. 1D).
Fig. 1 illustrates the distribution of MCC (Mathew Correlation Coefficient) values of each model. A) IPI (CLL-IPI features) versus MUT (CLL-IPI plus recurrent mutations features), B) IPI versus BL (CLL-IPI features plus (para)clinical baseline features), C) MUT versus BL, D) BL versus FULL (All the features)
Conclusion
We here demonstrate that including data on recurrent mutations and (para)clinical features on top of CLL- IPI can improve the predictive performance in a Machine Learning model predicting CLL treatment. Furthermore, a data driven selection of the optimal Machine Learning algorithm improves the predictive power. Upon confirmation of these findings in a blinded internal validation set and in external cohorts, these data indicate that combined modelling of multidimensional OMICs and (para)clinical data can pave the way for truly personalized management of CLL.
Keyword(s): Chronic lymphocytic leukemia, Clinical data, Mutation, Treatment
Abstract: EP602
Type: E-Poster Presentation
Session title: Chronic lymphocytic leukemia and related disorders - Biology & Translational Research
Background
The international prognostic index for patients with chronic lymphocytic leukemia (CLL-IPI) is the current standard for prognostication. We previously improved the CLL-IPI by 1) using a Treatment and Infection Model (CLL-TIM) and 2) by the number of signaling pathways impaired by recurrent mutations. In this study, we compare the performance of Machine Learning models trained on CLL-IPI variables plus baseline (para)clinical variables with CLL-IPI plus recurrent mutations in patients with newly diagnosed CLL.
Aims
1) Investigating the predictive performance of CLL-IPI variables, recurrent gene mutations, and (para)clinical variables for Treatment at time of CLL diagnosis.
2) Studying the impact of data-driven selection of the Machine Learning algorithm as well as the interaction between data variables and the algorithms.
Methods
In a cohort consisting of 314 patients with newly diagnosed CLL, CLL-IPI variables, recurrent gene mutations, and (para)clinical variables were extracted and used as features in a predictive framework in which a variety of Machine Learning algorithms (Linear SVM, AdaBoost, CatBoost, XGBoost, LightGBM, RandomForest, ExtraTrees, and Bagging) were used to predict CLL treatment as the outcome.
By using different combinations of feature sets, four models were defined as following:
- CLL-IPI features only (IPI),
- CLL-IPI features plus recurrent mutations (MUT),
- CLL-IPI features plus (para)clinical baseline features (BL),
- All the above features (FULL).
Patients were randomly split into a 60% train set and a 40% blinded validation set. The train set was further split 40 times into training set (75%) and test set (25%) to perform hypothesis testing, whereas the validation is still blinded. We here report the preliminary results on the train set.
Results
The preliminary results showed that adding either data on recurrent mutations (MUT) or (para)clinical baseline data (BL) improved the predictive performance significantly compared to the CLL-IPI only (Fig. 1A and 1B). Comparing the BL and MUT models, showed that adding baseline (para)clinical features (BL) predicted CLL treatment better than adding recurrent mutations (MUT) (Fig. 1C). As expected, including all the three feature sets (FULL) outperformed the BL model. For all four hypotheses, the Bagging algorithm achieved the best performance (Fig. 1D).
Fig. 1 illustrates the distribution of MCC (Mathew Correlation Coefficient) values of each model. A) IPI (CLL-IPI features) versus MUT (CLL-IPI plus recurrent mutations features), B) IPI versus BL (CLL-IPI features plus (para)clinical baseline features), C) MUT versus BL, D) BL versus FULL (All the features)
Conclusion
We here demonstrate that including data on recurrent mutations and (para)clinical features on top of CLL- IPI can improve the predictive performance in a Machine Learning model predicting CLL treatment. Furthermore, a data driven selection of the optimal Machine Learning algorithm improves the predictive power. Upon confirmation of these findings in a blinded internal validation set and in external cohorts, these data indicate that combined modelling of multidimensional OMICs and (para)clinical data can pave the way for truly personalized management of CLL.
Keyword(s): Chronic lymphocytic leukemia, Clinical data, Mutation, Treatment