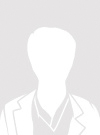
Contributions
Abstract: EP582
Type: E-Poster Presentation
Session title: Bone marrow failure syndromes incl. PNH - Biology & Translational Research
Background
Diamond Blackfan anemia (DBA) is a rare inherited bone marrow failure syndrome characterized by erythroid hypoplasia, congenital malformations, growth defects and an increased risk of developing malignancies. From a clinical and genetic perspective the disorder is highly heterogeneous, and clear genotype-phenotype correlations are absent.
Despite advances in our understanding of the genetic basis, the pathophysiology of DBA is still not fully understood. One of the major unresolved issues is how RP gene mutations result in the specific erythroid defect that characterizes DBA.
Establishing the diagnosis of DBA can be particularly difficult since, in contrast to other IBMFS, no validated functional screening tests exists. Furthermore, the clinical presentation is highly heterogeneous, even within families with identical molecular defects. Currently, the diagnosis relies on consensus criteria and exclusion of other IBMFs and causes of anemia.
In order to improve the diagnostic evaluation of DBA as well as our understanding of phenotypic heterogeneity and clinical severity, novel functional approaches are needed.
In this study we explore the potential of untargeted metabolomics on dried blood spots (DBS) to diagnose DBA.
Aims
Defining a metabolic signature for DBA from DBS, could 1. Contribute to improvement of the diagnostic evaluation, and 2. Increase our understanding of phenotypic heterogeneity and treatment response.
Methods
Untargeted metabolomics was applied on DBS samples obtained from 18 DBA patients and 40 healthy controls by direct infusion high resolution mass spectrometry using a previously established protocol (1,2). Statistical analyses were performed in MetaboAnalyst and predictive modelling was executed within R-software.
Results
A total of 1752 unique metabolite features were identified in the respective DBS samples from patients and controls. Distinct metabolic profiles were identified by principal component analysis and partial least squares discriminant analysis (Figure 1A-B).
Distinctive metabolites in this metabolic fingerprint include methylmalonylcarnitine, inosine and threonic acid (Figure 1C). The metabolic fingerprint of DBA was incorporated into a machine learning algorithm, and a binary classification model was constructed using a training set. The model showed high performance characteristics (average accuracy 93.5%) and correct prediction of class was observed for all controls (n=12) and all but one patient (n=4/5) from the validation or ‘test’ set (accuracy 94%) (Figure 1D). Importantly, in patients with congenital dyserythropoietic anemia (CDA), an erythroid disorder with overlapping features, we observed a distinct metabolic profile, indicating the disease specificity of the DBA fingerprint and underlining its diagnostic potential (Figure 1E). Furthermore, when exploring phenotypic heterogeneity, DBA treatment subgroups yielded discrete differences in metabolic profiles, which could hold future potential in understanding therapy responses (Figure 1F).
Conclusion
In this study we performed untargeted metabolomics in dried blood spots in a relatively large cohort of DBA patients with diverse genotypes and clinical phenotypes. We report here for the first time a specific metabolic fingerprint for DBA. By incorporating this fingerprint into a machine learning algorithm we demonstrate that untargeted metabolomics in dried blood spots is a promising new diagnostic tool for DBA.
Keyword(s): Bone marrow failure, Corticosteroids, Diagnosis, Diamond-Blackfan anemia
Abstract: EP582
Type: E-Poster Presentation
Session title: Bone marrow failure syndromes incl. PNH - Biology & Translational Research
Background
Diamond Blackfan anemia (DBA) is a rare inherited bone marrow failure syndrome characterized by erythroid hypoplasia, congenital malformations, growth defects and an increased risk of developing malignancies. From a clinical and genetic perspective the disorder is highly heterogeneous, and clear genotype-phenotype correlations are absent.
Despite advances in our understanding of the genetic basis, the pathophysiology of DBA is still not fully understood. One of the major unresolved issues is how RP gene mutations result in the specific erythroid defect that characterizes DBA.
Establishing the diagnosis of DBA can be particularly difficult since, in contrast to other IBMFS, no validated functional screening tests exists. Furthermore, the clinical presentation is highly heterogeneous, even within families with identical molecular defects. Currently, the diagnosis relies on consensus criteria and exclusion of other IBMFs and causes of anemia.
In order to improve the diagnostic evaluation of DBA as well as our understanding of phenotypic heterogeneity and clinical severity, novel functional approaches are needed.
In this study we explore the potential of untargeted metabolomics on dried blood spots (DBS) to diagnose DBA.
Aims
Defining a metabolic signature for DBA from DBS, could 1. Contribute to improvement of the diagnostic evaluation, and 2. Increase our understanding of phenotypic heterogeneity and treatment response.
Methods
Untargeted metabolomics was applied on DBS samples obtained from 18 DBA patients and 40 healthy controls by direct infusion high resolution mass spectrometry using a previously established protocol (1,2). Statistical analyses were performed in MetaboAnalyst and predictive modelling was executed within R-software.
Results
A total of 1752 unique metabolite features were identified in the respective DBS samples from patients and controls. Distinct metabolic profiles were identified by principal component analysis and partial least squares discriminant analysis (Figure 1A-B).
Distinctive metabolites in this metabolic fingerprint include methylmalonylcarnitine, inosine and threonic acid (Figure 1C). The metabolic fingerprint of DBA was incorporated into a machine learning algorithm, and a binary classification model was constructed using a training set. The model showed high performance characteristics (average accuracy 93.5%) and correct prediction of class was observed for all controls (n=12) and all but one patient (n=4/5) from the validation or ‘test’ set (accuracy 94%) (Figure 1D). Importantly, in patients with congenital dyserythropoietic anemia (CDA), an erythroid disorder with overlapping features, we observed a distinct metabolic profile, indicating the disease specificity of the DBA fingerprint and underlining its diagnostic potential (Figure 1E). Furthermore, when exploring phenotypic heterogeneity, DBA treatment subgroups yielded discrete differences in metabolic profiles, which could hold future potential in understanding therapy responses (Figure 1F).
Conclusion
In this study we performed untargeted metabolomics in dried blood spots in a relatively large cohort of DBA patients with diverse genotypes and clinical phenotypes. We report here for the first time a specific metabolic fingerprint for DBA. By incorporating this fingerprint into a machine learning algorithm we demonstrate that untargeted metabolomics in dried blood spots is a promising new diagnostic tool for DBA.
Keyword(s): Bone marrow failure, Corticosteroids, Diagnosis, Diamond-Blackfan anemia