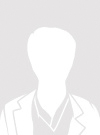
Contributions
Abstract: EP483
Type: E-Poster Presentation
Session title: Acute myeloid leukemia - Clinical
Background
Residual disease detection and monitoring by flow cytometry guides clinical physicians to modify treatment strategies according to patient’s risk profile. Current flow cytometry analysis approach is based on manual interpretation, which is relatively labor-intensive and time-consuming.
Aims
We propose to use machine learning algorithm for residual disease percentage (RDP) classification by using clinical flow cytometry (FC) data.
Methods
Retrospective clinical FC data of AML patients, as well as demographic data (age & gender) were collected from National Taiwan University Hospital. From 2013 to 2016, a total of 487 FC data positive for residual disease from 249 AML patients were enrolled in this study. There are 81 FC data with RDP within 0.01 to less than 1%, and 406 FC data with RDP greater than or equal to 1%. The median age at flow cytometry test performed was 51.8 years old.
Our proposed machine learning framework includes a phenotype representation learning paradigm and a classification model. To derive the phenotype representation, we trained a multivariate Gaussian Mixture Model (GMM) on the 38-dimension FC data to capture the training data distribution and characteristics in a probabilistic unsupervised manner. Then, a Fisher-scoring method derived from the differential of the learned GMM parameters was used to vectorize each sample as a high dimensional representation. This Fisher vectorization method transformed samples to a high dimensional feature space as phenotype vectors, which were finally fed into the random forest (RF) classifier. To alleviate the negative effects of imbalance classes in RDP identification tasks, we applied synthetic minority oversampling technique (SMOTE) algorithm which augmented the minority class by linearly interpolating synthetic samples from existing samples in the minority class. We train RF models for original fisher vectorization feature set and oversampled set separately to discriminate the RDP classes. The algorithm is evaluated by randomly divided 5-fold cross validation which separates 80% data for training and 20% for testing.
Results
The accuracy (ACC) and area under the ROC curve (AUC) of RDP prediction models achieved 0.897 and 0.934, respectively (Table 1a). Around 91.9% of those FC data with RDP greater than or equal to 1%, and 79.0% of those FC data with RDP within 0.01 to less than 1% are correctly classified when using oversampled set (Table 1b).
Conclusion
This study demonstrated the potential of machine learning algorithm used in RDP prediction in patient with AML. Further study with larger cohorts or different data sources are needed to validate this machine learning based prediction model as a clinical support tool to assist physicians in clinical decision making.
Keyword(s): Acute myeloid leukemia, Automation, Flow cytometry, Minimal residual disease (MRD)
Abstract: EP483
Type: E-Poster Presentation
Session title: Acute myeloid leukemia - Clinical
Background
Residual disease detection and monitoring by flow cytometry guides clinical physicians to modify treatment strategies according to patient’s risk profile. Current flow cytometry analysis approach is based on manual interpretation, which is relatively labor-intensive and time-consuming.
Aims
We propose to use machine learning algorithm for residual disease percentage (RDP) classification by using clinical flow cytometry (FC) data.
Methods
Retrospective clinical FC data of AML patients, as well as demographic data (age & gender) were collected from National Taiwan University Hospital. From 2013 to 2016, a total of 487 FC data positive for residual disease from 249 AML patients were enrolled in this study. There are 81 FC data with RDP within 0.01 to less than 1%, and 406 FC data with RDP greater than or equal to 1%. The median age at flow cytometry test performed was 51.8 years old.
Our proposed machine learning framework includes a phenotype representation learning paradigm and a classification model. To derive the phenotype representation, we trained a multivariate Gaussian Mixture Model (GMM) on the 38-dimension FC data to capture the training data distribution and characteristics in a probabilistic unsupervised manner. Then, a Fisher-scoring method derived from the differential of the learned GMM parameters was used to vectorize each sample as a high dimensional representation. This Fisher vectorization method transformed samples to a high dimensional feature space as phenotype vectors, which were finally fed into the random forest (RF) classifier. To alleviate the negative effects of imbalance classes in RDP identification tasks, we applied synthetic minority oversampling technique (SMOTE) algorithm which augmented the minority class by linearly interpolating synthetic samples from existing samples in the minority class. We train RF models for original fisher vectorization feature set and oversampled set separately to discriminate the RDP classes. The algorithm is evaluated by randomly divided 5-fold cross validation which separates 80% data for training and 20% for testing.
Results
The accuracy (ACC) and area under the ROC curve (AUC) of RDP prediction models achieved 0.897 and 0.934, respectively (Table 1a). Around 91.9% of those FC data with RDP greater than or equal to 1%, and 79.0% of those FC data with RDP within 0.01 to less than 1% are correctly classified when using oversampled set (Table 1b).
Conclusion
This study demonstrated the potential of machine learning algorithm used in RDP prediction in patient with AML. Further study with larger cohorts or different data sources are needed to validate this machine learning based prediction model as a clinical support tool to assist physicians in clinical decision making.
Keyword(s): Acute myeloid leukemia, Automation, Flow cytometry, Minimal residual disease (MRD)