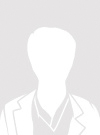
Contributions
Abstract: EP399
Type: E-Poster Presentation
Session title: Acute myeloid leukemia - Biology & Translational Research
Background
Although high rates of initial responders to cancer therapies are observed, relapse and metastasis are the most frequent causes of cancer-related death. Providing insights into cancer cell populations, and elucidating biological mechanisms of relapse is necessary for tailoring more effective treatment options. This is also apparent in Acute Myeloid Leukaemia (AML), a highly heterogeneous aggressive blood cancer with poor overall survival. Hence, there is a major challenge that could be addressed using high-throughput single-cell technologies combined with effective bioinformatics tools.
Aims
We hypothesised that characterizing hematopoietic cells in AML, and investigating the strength of signalling interactions between proteins at time of diagnosis, could identify predictors of survival.
Methods
To investigate our hypothesis, we performed CyTOF analysis on peripheral blood samples collected from 45 AML patients. The samples were stained with an antibody panel consisting of 21 surface and 15 intracellular markers, allowing for deep phenotypic profiling as well as monitoring of signalling pathways. To improve phenotypic characterisation of hematopoietic cells, a gating strategy was implemented using data from seven healthy donors. Next, Linear Discriminant Analysis allowed us to align cancer cells to their closest healthy cell phenotypes, resulting in a single-cell hematopoietic classifier. To alleviate the challenge of modelling complex single-cell data, Conditional Density Resampled Estimate of Mutual Information (DREMI) was utilised. DREMI quantifies the information transfer between pairs of proteins measured with single-cell resolution. Application of Machine Learning (ML) on DREMI-derived features combined with Feature Selection, allowed us to develop cell type specific models for survival, and to rank the most informative signalling interactions.
Results
By applying ML to DREMI-derived features extracted from different hematopoietic cell populations, we constructed cell type specific models for survival that were validated twice, with repeated cross-validation (n=38) and an independent validation cohort (n=7). Our analysis suggested that signalling interactions of cancer cells aligned to Megakaryocyte-Erythroid Progenitor (MEP) cells defined on the basis of CD45-low/CD34+/CD38+/CD123-low markers at time of diagnosis, predicted clinical outcome with an average performance of 0.83 Area Under the Curve, 0.78 Balanced Accuracy, 0.86 Positive Predictive Value and 0.82 F1 score. Our analysis also suggested that three signalling interactions of MEP cells between, pCREB-pSTAT5, pSTAT1-pSTAT5 and pHistone3-pSTAT5 were the most informative predictors. Retrospective analysis of both training/testing and validations cohorts (n=45), using Cox proportional-hazards modelling and Kaplan-Meier survival analysis, re-confirmed the potentials of the identified interactions to predict clinical outcome. Finally, our framework allowed us to investigate the dynamics of the identified interactions, using paired CyTOF samples collected 4 and 24 hours after chemotherapy initiation.
Conclusion
We presented a novel data-drive approach that leverages hematopoietic single-cell classification with ML modelling of signalling interactions to predict survival. In this way, our work provides direct associations between cell phenotypes, signalling states and clinical outcome. Although, the developed computational framework, has been specifically designed and applied for the analysis of signalling dynamics in AML, it can be applied to other cancer types as well.
Keyword(s): Relapsed acute myeloid leukemia, Signal transduction
Abstract: EP399
Type: E-Poster Presentation
Session title: Acute myeloid leukemia - Biology & Translational Research
Background
Although high rates of initial responders to cancer therapies are observed, relapse and metastasis are the most frequent causes of cancer-related death. Providing insights into cancer cell populations, and elucidating biological mechanisms of relapse is necessary for tailoring more effective treatment options. This is also apparent in Acute Myeloid Leukaemia (AML), a highly heterogeneous aggressive blood cancer with poor overall survival. Hence, there is a major challenge that could be addressed using high-throughput single-cell technologies combined with effective bioinformatics tools.
Aims
We hypothesised that characterizing hematopoietic cells in AML, and investigating the strength of signalling interactions between proteins at time of diagnosis, could identify predictors of survival.
Methods
To investigate our hypothesis, we performed CyTOF analysis on peripheral blood samples collected from 45 AML patients. The samples were stained with an antibody panel consisting of 21 surface and 15 intracellular markers, allowing for deep phenotypic profiling as well as monitoring of signalling pathways. To improve phenotypic characterisation of hematopoietic cells, a gating strategy was implemented using data from seven healthy donors. Next, Linear Discriminant Analysis allowed us to align cancer cells to their closest healthy cell phenotypes, resulting in a single-cell hematopoietic classifier. To alleviate the challenge of modelling complex single-cell data, Conditional Density Resampled Estimate of Mutual Information (DREMI) was utilised. DREMI quantifies the information transfer between pairs of proteins measured with single-cell resolution. Application of Machine Learning (ML) on DREMI-derived features combined with Feature Selection, allowed us to develop cell type specific models for survival, and to rank the most informative signalling interactions.
Results
By applying ML to DREMI-derived features extracted from different hematopoietic cell populations, we constructed cell type specific models for survival that were validated twice, with repeated cross-validation (n=38) and an independent validation cohort (n=7). Our analysis suggested that signalling interactions of cancer cells aligned to Megakaryocyte-Erythroid Progenitor (MEP) cells defined on the basis of CD45-low/CD34+/CD38+/CD123-low markers at time of diagnosis, predicted clinical outcome with an average performance of 0.83 Area Under the Curve, 0.78 Balanced Accuracy, 0.86 Positive Predictive Value and 0.82 F1 score. Our analysis also suggested that three signalling interactions of MEP cells between, pCREB-pSTAT5, pSTAT1-pSTAT5 and pHistone3-pSTAT5 were the most informative predictors. Retrospective analysis of both training/testing and validations cohorts (n=45), using Cox proportional-hazards modelling and Kaplan-Meier survival analysis, re-confirmed the potentials of the identified interactions to predict clinical outcome. Finally, our framework allowed us to investigate the dynamics of the identified interactions, using paired CyTOF samples collected 4 and 24 hours after chemotherapy initiation.
Conclusion
We presented a novel data-drive approach that leverages hematopoietic single-cell classification with ML modelling of signalling interactions to predict survival. In this way, our work provides direct associations between cell phenotypes, signalling states and clinical outcome. Although, the developed computational framework, has been specifically designed and applied for the analysis of signalling dynamics in AML, it can be applied to other cancer types as well.
Keyword(s): Relapsed acute myeloid leukemia, Signal transduction