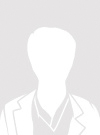
Contributions
Abstract: EP331
Type: E-Poster Presentation
Session title: Acute lymphoblastic leukemia - Biology & Translational Research
Background
A new provisional entity ‘B-lymphoblastic leukaemia/lymphoma, BCR-ABL1-like’ has been incorporated in the revised edition of WHO classification (2017). Authors across globe employ two approaches namely Microarray Gene Expression Profiling (GEP) or targeted Next-Generation Sequencing (NGS) and LDA (low-density array) card for its detection. In India, both these techniques are either not available or are prohibitively expensive. Therefore, we planned to perform identification and molecular characterisation of Indian BCR-ABL1-like ALL patients, using a different approach.
Aims
To identify BCR-ABL1-like ALL cases using two different platforms, followed by its molecular characterization.
Methods
Flowcytometric Immunophenotying (FCM-IP) and multiplex RT-PCR were performed on 611 B-lineage ALL cases to detect BCR-ABL1 fusion transcripts. Further, microarray profiling of 12 BCR-ABL1-positive cases was performed using Affymetrix U133 Plus 2.0 array. A total of 96 B-ALL cases were subjected to a targeted panel of 50 genes using nCounter GEP. Furthermore, we performed a deep molecular analysis in various genetic subgroups of identified BCR-ABL1-like ALL cases using nCounter GEP, quantitative Real time (RQ)-PCR & digital droplet PCR (DD-PCR). Lastly, we performed quantification of chosen 8 best differentially expressed (DE) genes on 551 B-ALL cases, to make a logistic regression model for its rapid detection.
Results
Multiplex RT-PCR assay revealed BCR-ABL1 transcripts in 17.67% (108/611) cases. Global transcriptome profiling of 12 BCR-ABL1 RNA transcripts revealed a total of 1574 as DE genes. DE genes were further filtered through hierarchical clustering analysis and a total of 45 DE genes were identified. Based on previous literature and regression coefficient values, 15 best classifier genes were selected using penalized logistic regression. Out of 78 examined BCR-ABL1-negative cases, 33 (42.30%) cases were clustered together with 15 BCR-ABL1-positive cases and were categorised as BCR-ABL1-like ALL cases in principal component analysis. Further, we categorised CRLF2 into two categories; high CRLF2 cases 5/33 (15.15%) & low 28/33 (84.84%) among BCR-ABL1-like ALL cases. JAK2 exon 16 was sequenced in 5 CRLF2 high cases and we found 2 missense variants, R683S and P694L, by Sanger sequencing. The average log fold change of -1.105 & -4.574 was seen in P2YR8 & CSF2RA respectively by RQ-PCR. In CRLF2 low expressing cases, the average log fold change of 12 kinase genes showed -1.11 in CENPC, -0.77 FOXP1, -0.20 NUP153, 1.12 RCSD1, 0.76 PAX5, -0.19 FLT3, -4.41 EPOR, -4.01 ILR2B, -1.58 CSFIR -3.43 PDGRFB, -5.98 NTRK3 and -1.99 ZNF274. The average log fold change of IKZF1 in 78 BCR-ABL1-negative cases was found to be 10.93 in nCounter GEP and 1.07 in RQ-PCR. We found the CNV incidence of 8.33% (9/108) in IKZF1 (4-7 deletion) gene and the ratio of IKZF1 deletion ranged from 6.14 to 69.8% using DD-PCR. Further, 8 genes were selected and quantified in 511 BCR-ABL1-negative cases and we found 104/551 (18.87%) BCR-ABL1-like ALL cases using RQ-PCR. Further, we created a dynamic nomogram using logistic regression based classifier for its rapid detection.
Conclusion
We have reported the incidence and devised a logistic regression based simple, efficient, cost-effective classifier for the rapid detection of BCR-ABL1 like ALL cases for the first time; which is likely to be suitable for developing countries. It is very important to detect BCR-ABL1-like ALL cases, so as to provide these patients the benefits of treatment stratification (including desirable TKI therapy) and prognostication.
Keyword(s): Acute lymphoblastic leukemia, B cell acute lymphoblastic leukemia, Lymphoid malignancy
Abstract: EP331
Type: E-Poster Presentation
Session title: Acute lymphoblastic leukemia - Biology & Translational Research
Background
A new provisional entity ‘B-lymphoblastic leukaemia/lymphoma, BCR-ABL1-like’ has been incorporated in the revised edition of WHO classification (2017). Authors across globe employ two approaches namely Microarray Gene Expression Profiling (GEP) or targeted Next-Generation Sequencing (NGS) and LDA (low-density array) card for its detection. In India, both these techniques are either not available or are prohibitively expensive. Therefore, we planned to perform identification and molecular characterisation of Indian BCR-ABL1-like ALL patients, using a different approach.
Aims
To identify BCR-ABL1-like ALL cases using two different platforms, followed by its molecular characterization.
Methods
Flowcytometric Immunophenotying (FCM-IP) and multiplex RT-PCR were performed on 611 B-lineage ALL cases to detect BCR-ABL1 fusion transcripts. Further, microarray profiling of 12 BCR-ABL1-positive cases was performed using Affymetrix U133 Plus 2.0 array. A total of 96 B-ALL cases were subjected to a targeted panel of 50 genes using nCounter GEP. Furthermore, we performed a deep molecular analysis in various genetic subgroups of identified BCR-ABL1-like ALL cases using nCounter GEP, quantitative Real time (RQ)-PCR & digital droplet PCR (DD-PCR). Lastly, we performed quantification of chosen 8 best differentially expressed (DE) genes on 551 B-ALL cases, to make a logistic regression model for its rapid detection.
Results
Multiplex RT-PCR assay revealed BCR-ABL1 transcripts in 17.67% (108/611) cases. Global transcriptome profiling of 12 BCR-ABL1 RNA transcripts revealed a total of 1574 as DE genes. DE genes were further filtered through hierarchical clustering analysis and a total of 45 DE genes were identified. Based on previous literature and regression coefficient values, 15 best classifier genes were selected using penalized logistic regression. Out of 78 examined BCR-ABL1-negative cases, 33 (42.30%) cases were clustered together with 15 BCR-ABL1-positive cases and were categorised as BCR-ABL1-like ALL cases in principal component analysis. Further, we categorised CRLF2 into two categories; high CRLF2 cases 5/33 (15.15%) & low 28/33 (84.84%) among BCR-ABL1-like ALL cases. JAK2 exon 16 was sequenced in 5 CRLF2 high cases and we found 2 missense variants, R683S and P694L, by Sanger sequencing. The average log fold change of -1.105 & -4.574 was seen in P2YR8 & CSF2RA respectively by RQ-PCR. In CRLF2 low expressing cases, the average log fold change of 12 kinase genes showed -1.11 in CENPC, -0.77 FOXP1, -0.20 NUP153, 1.12 RCSD1, 0.76 PAX5, -0.19 FLT3, -4.41 EPOR, -4.01 ILR2B, -1.58 CSFIR -3.43 PDGRFB, -5.98 NTRK3 and -1.99 ZNF274. The average log fold change of IKZF1 in 78 BCR-ABL1-negative cases was found to be 10.93 in nCounter GEP and 1.07 in RQ-PCR. We found the CNV incidence of 8.33% (9/108) in IKZF1 (4-7 deletion) gene and the ratio of IKZF1 deletion ranged from 6.14 to 69.8% using DD-PCR. Further, 8 genes were selected and quantified in 511 BCR-ABL1-negative cases and we found 104/551 (18.87%) BCR-ABL1-like ALL cases using RQ-PCR. Further, we created a dynamic nomogram using logistic regression based classifier for its rapid detection.
Conclusion
We have reported the incidence and devised a logistic regression based simple, efficient, cost-effective classifier for the rapid detection of BCR-ABL1 like ALL cases for the first time; which is likely to be suitable for developing countries. It is very important to detect BCR-ABL1-like ALL cases, so as to provide these patients the benefits of treatment stratification (including desirable TKI therapy) and prognostication.
Keyword(s): Acute lymphoblastic leukemia, B cell acute lymphoblastic leukemia, Lymphoid malignancy