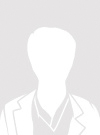
Contributions
Abstract: EP1181
Type: E-Poster Presentation
Session title: Quality of life, palliative care, ethics and health economics
Background
In the last 10 years, the amount of clinical research published in the field of oncology has grown dramatically due to accelerated pace of drug development and increased use of combination treatments. Concurrently, the problem of finding high-quality clinical research publications to develop evidence-based treatment plans for individual patients has become more challenging. Commonly used solutions primarily rely on bibliographic metadata and expert curation. Here, we describe a tool for fast automatic identification of clinically relevant publications that does not use the tags associated with the publication or curation.
Aims
Here, we describe a tool for fast automatic identification of clinically relevant publications that does not use the tags associated with the publication or curation.
Methods
We used a machine learning approach, trained on the titles of PubMed publications downloaded to the database through OncoTriage.com service. Papers predominantly describing clinical trials in hematological malignancies and clinical cases were used in the analysis. Balanced training data included texts cited in expert-curated sources to form a “relevant” dataset (i.e., high quality publications describing treatment of hematologic malignancy), and an “irrelevant” dataset that did not include data relevant to therapy. We used a Bayes approach with a binary classification. Briefly, 26,667 texts were processed to get a document-term matrix representation of both the training and the test set (80/20 split).
Results
Our model for “irrelevant” detection classified papers in the test dataset with AUC 0.859 accuracy (95%CI 0.853-0.865, p<0.0001), with sensitivity 0.93 and specificity 0.72. The balance of the model was biased towards the sensitivity. We speculate that our training dataset for the model was skewed towards publications describing clinical trials. Therefore, several clinically relevant categories of publications describing treatments were labeled as “irrelevant”. An expert examination of the false positives has revealed that these publications included therapy reviews, single center practices and observational studies that nonetheless are informative for clinical practice. We plan to address these drawbacks in future iterations of the model by incorporating supervised or reinforcement learning approaches. The interactive web app is available at https://luchinin.shinyapps.io/PubMed_Triage/
Conclusion
Machine learning is an effective approach for a large-scale automaticidentification of clinically relevant publications from a variety of databases, such asPubMed and conference abstracts. The use of machine learning techniques as one of the filtersto build a database tailored for clinicians. In the future tools using this database may help tominimize the time clinicians spend finding high-quality publications that fit their patient’s profile.In addition, it can be used as a clean-up step for getting a list of publications for further curationby the subject matter experts. Future work will extend this approach and may be integrated intodecision support systems and knowledge management databases.
Keyword(s): Hematological malignancy, Therapy
Abstract: EP1181
Type: E-Poster Presentation
Session title: Quality of life, palliative care, ethics and health economics
Background
In the last 10 years, the amount of clinical research published in the field of oncology has grown dramatically due to accelerated pace of drug development and increased use of combination treatments. Concurrently, the problem of finding high-quality clinical research publications to develop evidence-based treatment plans for individual patients has become more challenging. Commonly used solutions primarily rely on bibliographic metadata and expert curation. Here, we describe a tool for fast automatic identification of clinically relevant publications that does not use the tags associated with the publication or curation.
Aims
Here, we describe a tool for fast automatic identification of clinically relevant publications that does not use the tags associated with the publication or curation.
Methods
We used a machine learning approach, trained on the titles of PubMed publications downloaded to the database through OncoTriage.com service. Papers predominantly describing clinical trials in hematological malignancies and clinical cases were used in the analysis. Balanced training data included texts cited in expert-curated sources to form a “relevant” dataset (i.e., high quality publications describing treatment of hematologic malignancy), and an “irrelevant” dataset that did not include data relevant to therapy. We used a Bayes approach with a binary classification. Briefly, 26,667 texts were processed to get a document-term matrix representation of both the training and the test set (80/20 split).
Results
Our model for “irrelevant” detection classified papers in the test dataset with AUC 0.859 accuracy (95%CI 0.853-0.865, p<0.0001), with sensitivity 0.93 and specificity 0.72. The balance of the model was biased towards the sensitivity. We speculate that our training dataset for the model was skewed towards publications describing clinical trials. Therefore, several clinically relevant categories of publications describing treatments were labeled as “irrelevant”. An expert examination of the false positives has revealed that these publications included therapy reviews, single center practices and observational studies that nonetheless are informative for clinical practice. We plan to address these drawbacks in future iterations of the model by incorporating supervised or reinforcement learning approaches. The interactive web app is available at https://luchinin.shinyapps.io/PubMed_Triage/
Conclusion
Machine learning is an effective approach for a large-scale automaticidentification of clinically relevant publications from a variety of databases, such asPubMed and conference abstracts. The use of machine learning techniques as one of the filtersto build a database tailored for clinicians. In the future tools using this database may help tominimize the time clinicians spend finding high-quality publications that fit their patient’s profile.In addition, it can be used as a clean-up step for getting a list of publications for further curationby the subject matter experts. Future work will extend this approach and may be integrated intodecision support systems and knowledge management databases.
Keyword(s): Hematological malignancy, Therapy