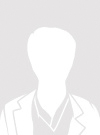
Contributions
Abstract: S310
Type: Oral Presentation
Session title: Patient's voice and health outcomes in hematology
Background
Cytomorphology is essential to quickly assess hematological neoplasms. Experienced personnel and short TAT of <24 hours are mandatory. Machine learning (ML) has proven its power in image classification. Following our pilot study (ASH 2020, Blood 136, # 195) we report on our first experiences with an ML-based blood cell differentiation tool in daily routine.
Aims
To evaluate feasibility in a prospective blinded trial in parallel to daily routine workflow, we registered the BELUGA study (ClinicalTrials.gov Identifier: NCT04466059) which includes a head-to-head-comparison between human investigators and the ML-based classifier regarding accuracy over all malignant/non-malignant cell types in peripheral blood.
Methods
We established an automated routine workflow including the capture of 500 digital cell images per blood smear in 40x oil magnification after focusing on regions of interest (Metafer Scanning System, MetaSystems, Altlussheim; Zeiss Axio Imager.Z2 microscope). Cells are classified by the ML-based deep neural network (DNN). Resulting data are compared to the independent microscopic cell differentiation done by hem specialists. With regard to future use in routine, misclassifications can be corrected by humans. Corrected images can be manually flagged to join a dataset for continuous retraining of the DNN.
Results
Since Jan 1, 2021 the BELUGA trial included 1,586 blood samples. The most common malignant diagnoses were: CLL, MPN, mature B-cell neoplasm, MDS, MBL, CML, AML and CMML. In all cases human investigators reach out to count 100-200 cells according to WHO guidelines. The addressed cell classes were PMN, lymphocytes, monocytes, eosinophils, basophils, bands, metamyelocytes, myelocytes, promyelocytes, myeloblasts, atypical/bilobulated promyelocytes in APL/APLv, hairy cells, atypical lymphocytes (potentially neoplastic), plasma cells, normoblasts, smudge cells, plus one garbage class for damaged cells. 155,159 cells were differentiated by humans (12 individuals, median experience in the field: 8 years), 769,347 cells (median 500 per sample) by the DNN model as described (ASH 2020, Blood 136, # 195). While human analysis took 5-10 minutes per sample, the DNN processes ~500 cells/images within 15 seconds after automated scanning time of 7 min. Probability of prediction was >0.95 for 46% of images, >0.90 was achieved for 55%. During the pilot study, we noticed misclassifications in cells representing neighbouring stages of maturation or in reactive vs. neoplastic lymphocytes. For refining the DNN, we reduced the number of images used for training, keeping only 10% (8,425 cells) of the original dataset. Our new version (V.2) tested now in the BELUGA study achieves an overall accuracy of 96.6% (vs. 89.6% in V.1) on this separate prospective test set. First evidence of clinical relevance could be seen in two cases with low count APL. The DNN (V.1) was able to detect atypical promyelocytes at a probability of ≥0.98.
Conclusion
We successfully integrated a fully automated process (cell scanning and classification by DNN) parallel to our diagnostic routine workflow without critical delay in TAT. The DNN outperformed human experts (investigation time, number of cells investigated) in a real-life setting and seems to also provide a higher sensitivity and specificity for critical cell types, thus being a helpful support for cytomorphologists. Such infrastructure being cloud-based and allowing access for uploading/classifying digital cell images could serve experts worldwide 24/7.
Keyword(s): Diagnosis, Leukemia, Phenotype
Abstract: S310
Type: Oral Presentation
Session title: Patient's voice and health outcomes in hematology
Background
Cytomorphology is essential to quickly assess hematological neoplasms. Experienced personnel and short TAT of <24 hours are mandatory. Machine learning (ML) has proven its power in image classification. Following our pilot study (ASH 2020, Blood 136, # 195) we report on our first experiences with an ML-based blood cell differentiation tool in daily routine.
Aims
To evaluate feasibility in a prospective blinded trial in parallel to daily routine workflow, we registered the BELUGA study (ClinicalTrials.gov Identifier: NCT04466059) which includes a head-to-head-comparison between human investigators and the ML-based classifier regarding accuracy over all malignant/non-malignant cell types in peripheral blood.
Methods
We established an automated routine workflow including the capture of 500 digital cell images per blood smear in 40x oil magnification after focusing on regions of interest (Metafer Scanning System, MetaSystems, Altlussheim; Zeiss Axio Imager.Z2 microscope). Cells are classified by the ML-based deep neural network (DNN). Resulting data are compared to the independent microscopic cell differentiation done by hem specialists. With regard to future use in routine, misclassifications can be corrected by humans. Corrected images can be manually flagged to join a dataset for continuous retraining of the DNN.
Results
Since Jan 1, 2021 the BELUGA trial included 1,586 blood samples. The most common malignant diagnoses were: CLL, MPN, mature B-cell neoplasm, MDS, MBL, CML, AML and CMML. In all cases human investigators reach out to count 100-200 cells according to WHO guidelines. The addressed cell classes were PMN, lymphocytes, monocytes, eosinophils, basophils, bands, metamyelocytes, myelocytes, promyelocytes, myeloblasts, atypical/bilobulated promyelocytes in APL/APLv, hairy cells, atypical lymphocytes (potentially neoplastic), plasma cells, normoblasts, smudge cells, plus one garbage class for damaged cells. 155,159 cells were differentiated by humans (12 individuals, median experience in the field: 8 years), 769,347 cells (median 500 per sample) by the DNN model as described (ASH 2020, Blood 136, # 195). While human analysis took 5-10 minutes per sample, the DNN processes ~500 cells/images within 15 seconds after automated scanning time of 7 min. Probability of prediction was >0.95 for 46% of images, >0.90 was achieved for 55%. During the pilot study, we noticed misclassifications in cells representing neighbouring stages of maturation or in reactive vs. neoplastic lymphocytes. For refining the DNN, we reduced the number of images used for training, keeping only 10% (8,425 cells) of the original dataset. Our new version (V.2) tested now in the BELUGA study achieves an overall accuracy of 96.6% (vs. 89.6% in V.1) on this separate prospective test set. First evidence of clinical relevance could be seen in two cases with low count APL. The DNN (V.1) was able to detect atypical promyelocytes at a probability of ≥0.98.
Conclusion
We successfully integrated a fully automated process (cell scanning and classification by DNN) parallel to our diagnostic routine workflow without critical delay in TAT. The DNN outperformed human experts (investigation time, number of cells investigated) in a real-life setting and seems to also provide a higher sensitivity and specificity for critical cell types, thus being a helpful support for cytomorphologists. Such infrastructure being cloud-based and allowing access for uploading/classifying digital cell images could serve experts worldwide 24/7.
Keyword(s): Diagnosis, Leukemia, Phenotype