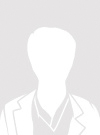
Contributions
Abstract: S178
Type: Oral Presentation
Session title: Basic and translational myeloma research
Background
Current clinical prognostication of newly diagnosed MM (NDMM) patients relies on clinical parameters and/or recurrent genetic changes, which mainly reflect early events in the development of MM. However, recent insights into the pathogenesis of MM highlighted genome/transcriptome editing through APOBEC/ADAR genes as well as inflammation as drivers for the onset and progression of MM.
Aims
To build a superior base editors/inflammation-based risk scoring system reflecting biological processes that drive the progression of MM.
Methods
We hypothesized that a prognostic score reflecting biological processes as well as clinical features is superior to the currently used classification systems for MM patients, such as ISS, R-ISS and the Mayo clinic classification. The Multiple Myeloma Research Foundation CoMMpass study genomics dataset, combining mRNA Seq and clinical data from more than 700 patients, allowed us to evaluate the prognostic value of demographic and clinical parameters, cytogenetics, and gene expression levels of ADAR and the APOBEC family as well as inflammation-modulating cytokines of MM patients. We calculated hazard ratios and Kaplan-Meier survival estimates for all extracted features. Combining clinical variables that were significantly associated with PFS and OS, we then applied machine learning approaches to identify the most accurate classification model to define a new risk score that is easy to compute and able to stratify NDMM patients more accurately than cytogenetics-based classifiers.
Results
Based on our machine learning models, we defined a weighted OS/PFS risk score (Editor-Inflammation score) based on expression of APOBEC2, APOBEC3B, APOBEC3C, APOBEC3D, ADAR1, IL10, IL11, IL27, TGFB1, TGFB2, as well as ß-2-microglobulin, hemoglobin, LDH and creatinine serum levels, that achieved the best classification outcome and showed superior performance compared to ISS and R-ISS. Of note, cytogenetic abnormalities did not proof relevant have therefore not been included in the EI score. Besides superior overall risk stratification, the EI score further allowed to identify subgroups of MM patients with very good prognosis and an “ultra high risk” group of patients who do not benefit from bone marrow transplantation maintenance therapy.
Conclusion
Our findings support the adoption of molecular biomarkers, reflecting dynamic biological processes rather than cytogenetics for a more accurate risk classification of MM. Considering that mRNA-seq is as cost effective as FISH and is being increasingly adopted by diagnostic centres, the EI score is a unique approach to shed light on underlying molecular mechanisms that drive disease progression and the development of true risk-adapted treatment strategies.
Keyword(s): Gene expression, Multiple myeloma, Prognosis
Abstract: S178
Type: Oral Presentation
Session title: Basic and translational myeloma research
Background
Current clinical prognostication of newly diagnosed MM (NDMM) patients relies on clinical parameters and/or recurrent genetic changes, which mainly reflect early events in the development of MM. However, recent insights into the pathogenesis of MM highlighted genome/transcriptome editing through APOBEC/ADAR genes as well as inflammation as drivers for the onset and progression of MM.
Aims
To build a superior base editors/inflammation-based risk scoring system reflecting biological processes that drive the progression of MM.
Methods
We hypothesized that a prognostic score reflecting biological processes as well as clinical features is superior to the currently used classification systems for MM patients, such as ISS, R-ISS and the Mayo clinic classification. The Multiple Myeloma Research Foundation CoMMpass study genomics dataset, combining mRNA Seq and clinical data from more than 700 patients, allowed us to evaluate the prognostic value of demographic and clinical parameters, cytogenetics, and gene expression levels of ADAR and the APOBEC family as well as inflammation-modulating cytokines of MM patients. We calculated hazard ratios and Kaplan-Meier survival estimates for all extracted features. Combining clinical variables that were significantly associated with PFS and OS, we then applied machine learning approaches to identify the most accurate classification model to define a new risk score that is easy to compute and able to stratify NDMM patients more accurately than cytogenetics-based classifiers.
Results
Based on our machine learning models, we defined a weighted OS/PFS risk score (Editor-Inflammation score) based on expression of APOBEC2, APOBEC3B, APOBEC3C, APOBEC3D, ADAR1, IL10, IL11, IL27, TGFB1, TGFB2, as well as ß-2-microglobulin, hemoglobin, LDH and creatinine serum levels, that achieved the best classification outcome and showed superior performance compared to ISS and R-ISS. Of note, cytogenetic abnormalities did not proof relevant have therefore not been included in the EI score. Besides superior overall risk stratification, the EI score further allowed to identify subgroups of MM patients with very good prognosis and an “ultra high risk” group of patients who do not benefit from bone marrow transplantation maintenance therapy.
Conclusion
Our findings support the adoption of molecular biomarkers, reflecting dynamic biological processes rather than cytogenetics for a more accurate risk classification of MM. Considering that mRNA-seq is as cost effective as FISH and is being increasingly adopted by diagnostic centres, the EI score is a unique approach to shed light on underlying molecular mechanisms that drive disease progression and the development of true risk-adapted treatment strategies.
Keyword(s): Gene expression, Multiple myeloma, Prognosis