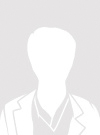
Contributions
Abstract: PB1806
Type: Publication Only
Session title: Thalassemias
Background
An effective screening programme to detect Thalassemia carriers is a vital part of Thalassemia prevention. There are many challenges to an effective screening programme,especially in low-resource settings. Considering alpha-thalassemia, genetic testing is needed for a confirmatory diagnosis of a carrier, which is expensive and not widely available.
In this era of big data and exponentially increasing computing power, machine learning (ML) is being increasingly used in a range of prognostic and diagnostic medical tasks to overcome domain-specific challenges.
Aims
This study attempted to apply modern machine learning algorithms to alpha-thalassemia screening data, and develop a cost-effective and time-saving ML tool which can accurately predict the alpha thalassemia carrier state using simple blood tests. This tool can then be used in circumstances where there are constraints to genetic testing, and can act as a complimentary diagnostic tool which can identify high risk individuals who need to undergo confirmatory genetic testing.
Methods
Ethical clearance was obtained from the Ethics Review Committee of Postgraduate Institute of Medicine, University of Colombo. A database of 288 cases from the Human Genetics Unit (HGU) of the Faculty of Medicine, Colombo was used to train and test the ML tool. Three different models were formulated and tested on a validation set. Random Forest Algorithm, and an Artificial Neural Network (ANN) with four hidden layers were used to train each of the models. The relative performances of the algorithms on each model were compared in terms of overall accuracy and F1 score, which is a measure of sensitivity and positive predictive value.
Results
Dataset included predominantly alpha-thalassemia carriers (n = 149), but also individuals with normal red cell indices (n = 55), iron deficiency anaemia (n = 21) and beta-thalassemia carriers (n = 17). Model 1 was trained to differentiate between alpha-thalassemia carriers and normal individuals, and the Random Forest outperformed the ANN with an Accuracy of 93.5% and an F1 score of 87.5%. Model 2 differentiated between alpha-thalassemia silent carriers and alpha-thalassemia traits, and the Random Forest again showed a superior performance with an accuracy of 88.8% and an F1 score of 86.3%. Both models showed a satisfactory performance in all categories in the confusion matrix which plotted the true labels against predicted labels. Model 3 was developed to differentiate between thalassemia carriers, iron deficiency and normal individuals. Although ANN showed a superior performance with an overall accuracy and F1 score of 83.3% on model 3, the model only correctly classified one of the three iron deficiency cases in the validation set.
Conclusion
Model 1 and 2 showed satisfactory performance, while model 3 did not. Model 1 and 2 can be combined to a diagnostic tool to identify alpha-thalassemia carrier states. The tool could be used in situations where there are constraints to genetic testing after being validated prospectively with a larger dataset.
Keyword(s): Hemoglobin variants, Hemoglobinopathy, Screening, Thalassemia
Abstract: PB1806
Type: Publication Only
Session title: Thalassemias
Background
An effective screening programme to detect Thalassemia carriers is a vital part of Thalassemia prevention. There are many challenges to an effective screening programme,especially in low-resource settings. Considering alpha-thalassemia, genetic testing is needed for a confirmatory diagnosis of a carrier, which is expensive and not widely available.
In this era of big data and exponentially increasing computing power, machine learning (ML) is being increasingly used in a range of prognostic and diagnostic medical tasks to overcome domain-specific challenges.
Aims
This study attempted to apply modern machine learning algorithms to alpha-thalassemia screening data, and develop a cost-effective and time-saving ML tool which can accurately predict the alpha thalassemia carrier state using simple blood tests. This tool can then be used in circumstances where there are constraints to genetic testing, and can act as a complimentary diagnostic tool which can identify high risk individuals who need to undergo confirmatory genetic testing.
Methods
Ethical clearance was obtained from the Ethics Review Committee of Postgraduate Institute of Medicine, University of Colombo. A database of 288 cases from the Human Genetics Unit (HGU) of the Faculty of Medicine, Colombo was used to train and test the ML tool. Three different models were formulated and tested on a validation set. Random Forest Algorithm, and an Artificial Neural Network (ANN) with four hidden layers were used to train each of the models. The relative performances of the algorithms on each model were compared in terms of overall accuracy and F1 score, which is a measure of sensitivity and positive predictive value.
Results
Dataset included predominantly alpha-thalassemia carriers (n = 149), but also individuals with normal red cell indices (n = 55), iron deficiency anaemia (n = 21) and beta-thalassemia carriers (n = 17). Model 1 was trained to differentiate between alpha-thalassemia carriers and normal individuals, and the Random Forest outperformed the ANN with an Accuracy of 93.5% and an F1 score of 87.5%. Model 2 differentiated between alpha-thalassemia silent carriers and alpha-thalassemia traits, and the Random Forest again showed a superior performance with an accuracy of 88.8% and an F1 score of 86.3%. Both models showed a satisfactory performance in all categories in the confusion matrix which plotted the true labels against predicted labels. Model 3 was developed to differentiate between thalassemia carriers, iron deficiency and normal individuals. Although ANN showed a superior performance with an overall accuracy and F1 score of 83.3% on model 3, the model only correctly classified one of the three iron deficiency cases in the validation set.
Conclusion
Model 1 and 2 showed satisfactory performance, while model 3 did not. Model 1 and 2 can be combined to a diagnostic tool to identify alpha-thalassemia carrier states. The tool could be used in situations where there are constraints to genetic testing after being validated prospectively with a larger dataset.
Keyword(s): Hemoglobin variants, Hemoglobinopathy, Screening, Thalassemia