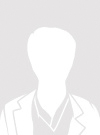
Contributions
Abstract: PB1645
Type: Publication Only
Session title: Myeloma and other monoclonal gammopathies - Clinical
Background
Multiple myeloma (MM) is one of the most common hematologic malignancies with more than 30,000 cases diagnosed in the US alone. This incurable disease is characterized by a high heterogeneity and complex pathophysiology. A tremendous progress in our ability to treat this disease has been made in the last two decades by introduction of new therapeutic modalities. Today, there are dozens of interventions that include radiation therapy, cell therapy and drug combinations available. Unfortunately, direct head-to-head comparisons between treatment regiments in MM are often available due to the high cost and time-consuming nature of additional clinical trials.
Aims
Here we describe a method for prediction of several (clinical) parameters of responses to MM therapy that uses statistical and machine learning approaches.
Methods
Clinical data including names of treatment options, response rates, progressive-free survival (PFS) and other parameters were extracted from scientific publications that described results of clinical trials and real-world practice in the management of patients with MM. We used a very good partial response (VGPR) and PFS as outcomes for the prediction. The prognosis of VGPR was calculated as follows: 1) we calculated inverse percentages for each clinical study and treatment method, 2) added all inverse percentages for a given treatment method to get an average rate, 3) VGPR rates between the two treatment options were compared by using Odds Ratio (OR). The prediction of PFS is based on a machine-learning algorithm - random forests for survival which uses VGPR, line of therapy and stem cell transplantation as predictors. All statistical analyses were done using R version 3.6.0. and the 'shiny', 'epiR', 'survminer', 'survival', 'ranger', 'ggplot2' packages.
Results
More than 300 publications were identified by a systematic literature review and 100 treatment options with a total of 40334 patients with MM were selected. The data from this corpus of publications were analyzed using open-source R software, and visualized via an interactive web app. The functions of the app include: 1) calculation VGPR or better for selected treatment options, 2) therapy response comparison with calculation of statistical significance (OR, p-value), 3) PFS prediction with interactive graphs of survival curves (Hazard ratio, p-value). The Harrell’s c-index for the model of PFS prediction is 0.81. The app is available at https://luchinin.shinyapps.io/MM_Therapy_Analyzer/. All treatment options are divided into two categories: “newly diagnosed MM” and “relapsed and refractory MM”. Current version of the web app provides an additional reference point for making a decision about the treatment strategy in patients with MM. The tool also helps to estimate efficacy of the therapy in relation to another for categories of clinical cases.
Conclusion
We developed an interactive digital tool and web app to aid selection of strategy for multiple myeloma treatment. We used machine learning and general statistics to calculate and predict key outcomes of therapy in patients with MM based on a systematic review of 300+ clinical publications. To the best of our knowledge, this is the first publication describing this approach combined with a user-friendly publicly available tool. Future work will extend this approach and explore the potential for integration into hematology clinical decision-support systems.
Keyword(s): Myeloma, Prognosis, Therapy
Abstract: PB1645
Type: Publication Only
Session title: Myeloma and other monoclonal gammopathies - Clinical
Background
Multiple myeloma (MM) is one of the most common hematologic malignancies with more than 30,000 cases diagnosed in the US alone. This incurable disease is characterized by a high heterogeneity and complex pathophysiology. A tremendous progress in our ability to treat this disease has been made in the last two decades by introduction of new therapeutic modalities. Today, there are dozens of interventions that include radiation therapy, cell therapy and drug combinations available. Unfortunately, direct head-to-head comparisons between treatment regiments in MM are often available due to the high cost and time-consuming nature of additional clinical trials.
Aims
Here we describe a method for prediction of several (clinical) parameters of responses to MM therapy that uses statistical and machine learning approaches.
Methods
Clinical data including names of treatment options, response rates, progressive-free survival (PFS) and other parameters were extracted from scientific publications that described results of clinical trials and real-world practice in the management of patients with MM. We used a very good partial response (VGPR) and PFS as outcomes for the prediction. The prognosis of VGPR was calculated as follows: 1) we calculated inverse percentages for each clinical study and treatment method, 2) added all inverse percentages for a given treatment method to get an average rate, 3) VGPR rates between the two treatment options were compared by using Odds Ratio (OR). The prediction of PFS is based on a machine-learning algorithm - random forests for survival which uses VGPR, line of therapy and stem cell transplantation as predictors. All statistical analyses were done using R version 3.6.0. and the 'shiny', 'epiR', 'survminer', 'survival', 'ranger', 'ggplot2' packages.
Results
More than 300 publications were identified by a systematic literature review and 100 treatment options with a total of 40334 patients with MM were selected. The data from this corpus of publications were analyzed using open-source R software, and visualized via an interactive web app. The functions of the app include: 1) calculation VGPR or better for selected treatment options, 2) therapy response comparison with calculation of statistical significance (OR, p-value), 3) PFS prediction with interactive graphs of survival curves (Hazard ratio, p-value). The Harrell’s c-index for the model of PFS prediction is 0.81. The app is available at https://luchinin.shinyapps.io/MM_Therapy_Analyzer/. All treatment options are divided into two categories: “newly diagnosed MM” and “relapsed and refractory MM”. Current version of the web app provides an additional reference point for making a decision about the treatment strategy in patients with MM. The tool also helps to estimate efficacy of the therapy in relation to another for categories of clinical cases.
Conclusion
We developed an interactive digital tool and web app to aid selection of strategy for multiple myeloma treatment. We used machine learning and general statistics to calculate and predict key outcomes of therapy in patients with MM based on a systematic review of 300+ clinical publications. To the best of our knowledge, this is the first publication describing this approach combined with a user-friendly publicly available tool. Future work will extend this approach and explore the potential for integration into hematology clinical decision-support systems.
Keyword(s): Myeloma, Prognosis, Therapy