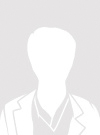
Contributions
Abstract: PB1826
Type: Publication Only
Background
Computational intelligence has been applied to a wide range of problems to assist in decision-making, especially artificial neural networks, fuzzy systems and powerful hybrid neuro-fuzzy approaches have already proven their strong potentials in medicine. Despite that, applications in hematology are still scarce.
Aims
In this study we have developed novel ANFIS neuro-fuzzy prognostic models, based on clinical and morphometric diagnostic data, to enable better prediction of complete cytogenetic response (CCgR) for patients with chronic myeloid leukemia.
Methods
This prospective study included a consecutive series of patients with chronic myeloid leukemia (CML) who were started on imatinib therapy. Analysis was performed with CCgR at 6, 12, and 18 months as the outcome variables. A total of 40 patients on imatinib therapy were included in the final analysis. Of these, 25 (62.5%), 29 (72.5%), and 32 (80%), respectively, achieved CCgR at 6, 12, and 18 months after initiation of imatinib. Computationally intelligent neuro-fuzzy models that were developed included EUTOS score on diagnosis and one of the following morphometric parameters: microvascular density, length of the minor axis, area or circularity of the blood vessel. Adaptive neuro-fuzzy systems represent a specific combination of artificial neural networks and fuzzy logic, thus combining the learning ability of artificial neural networks with the knowledge representation capability of fuzzy logic systems. ANFIS (Adaptive Neuro Fuzzy Inference System) consists of five layers of nodes (neurons), each of which performs a particular function on incoming signals as well as a set of parameters pertaining to this node. The basic architecture of ANFIS using hybrid learning algorithm is presented in Figure 1.
Results
All analysed patients have received imatinib mesylate as their first-line therapy for CML. Model predictions (0–1) for any individual patient were interpreted as probability of CCgR at 6, 12 or 18 months. The overall accuracy of the final model was determined by comparing the predicted values with the actual events. A probability cut-off point of 0.50 (50 %) was used to classify observations as events or non events, and patents were divided in training, validation and testing groups. Best performing ANFIS model, including EUTOS score and minor axis morphometric parameter was better than a model that includes only EUTOS score and regression model based on the same inputs. Overall model correct classification achieved for EUTOS, two input LR model and two input ANFIS model were respectively 75%, 75% and 77.5%, while areas under curve on ROC graphs were 0.776, 0.829 and 0.875 respectively.
Conclusion
The major finding of this study is that ANFIS models using the morphometric parameters, available at diagnosis of chronic phase of the CML, may improve prediction of CCgR at 6, 12 and 18 months on imatinib therapy, in comparison to the EUTOS score being the standard prognostic scoring system and regression models using the same inputs. Using neuro-fuzzy computationally intelligent ANFIS models with morphometric parameters in conjunction with EUTOS score improves prediction of CCgR. Validation on larger groups of patients is needed, but these findings indicate that neuro fuzzy models could aid in individual CML patient risk stratification.
Session topic: 8. Chronic myeloid leukemia - Clinical
Keyword(s): prognosis, imatinib, Chronic myeloid leukemia, Angiogenesis
Abstract: PB1826
Type: Publication Only
Background
Computational intelligence has been applied to a wide range of problems to assist in decision-making, especially artificial neural networks, fuzzy systems and powerful hybrid neuro-fuzzy approaches have already proven their strong potentials in medicine. Despite that, applications in hematology are still scarce.
Aims
In this study we have developed novel ANFIS neuro-fuzzy prognostic models, based on clinical and morphometric diagnostic data, to enable better prediction of complete cytogenetic response (CCgR) for patients with chronic myeloid leukemia.
Methods
This prospective study included a consecutive series of patients with chronic myeloid leukemia (CML) who were started on imatinib therapy. Analysis was performed with CCgR at 6, 12, and 18 months as the outcome variables. A total of 40 patients on imatinib therapy were included in the final analysis. Of these, 25 (62.5%), 29 (72.5%), and 32 (80%), respectively, achieved CCgR at 6, 12, and 18 months after initiation of imatinib. Computationally intelligent neuro-fuzzy models that were developed included EUTOS score on diagnosis and one of the following morphometric parameters: microvascular density, length of the minor axis, area or circularity of the blood vessel. Adaptive neuro-fuzzy systems represent a specific combination of artificial neural networks and fuzzy logic, thus combining the learning ability of artificial neural networks with the knowledge representation capability of fuzzy logic systems. ANFIS (Adaptive Neuro Fuzzy Inference System) consists of five layers of nodes (neurons), each of which performs a particular function on incoming signals as well as a set of parameters pertaining to this node. The basic architecture of ANFIS using hybrid learning algorithm is presented in Figure 1.
Results
All analysed patients have received imatinib mesylate as their first-line therapy for CML. Model predictions (0–1) for any individual patient were interpreted as probability of CCgR at 6, 12 or 18 months. The overall accuracy of the final model was determined by comparing the predicted values with the actual events. A probability cut-off point of 0.50 (50 %) was used to classify observations as events or non events, and patents were divided in training, validation and testing groups. Best performing ANFIS model, including EUTOS score and minor axis morphometric parameter was better than a model that includes only EUTOS score and regression model based on the same inputs. Overall model correct classification achieved for EUTOS, two input LR model and two input ANFIS model were respectively 75%, 75% and 77.5%, while areas under curve on ROC graphs were 0.776, 0.829 and 0.875 respectively.
Conclusion
The major finding of this study is that ANFIS models using the morphometric parameters, available at diagnosis of chronic phase of the CML, may improve prediction of CCgR at 6, 12 and 18 months on imatinib therapy, in comparison to the EUTOS score being the standard prognostic scoring system and regression models using the same inputs. Using neuro-fuzzy computationally intelligent ANFIS models with morphometric parameters in conjunction with EUTOS score improves prediction of CCgR. Validation on larger groups of patients is needed, but these findings indicate that neuro fuzzy models could aid in individual CML patient risk stratification.
Session topic: 8. Chronic myeloid leukemia - Clinical
Keyword(s): prognosis, imatinib, Chronic myeloid leukemia, Angiogenesis