CLINICAL DECISION SUPPORT SYSTEM (CDSS) BASED ON PATHWAYS-CONSTRAINED SOMATIC MUTATIONAL DATA INTEGRATION IN ACUTE MYELOID LEUKEMIA PERSONALIZED MEDICINE
(Abstract release date: 05/19/16)
EHA Library. Manfrini M. 06/09/16; 132477; E928
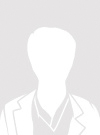
Prof. Marco Manfrini
Contributions
Contributions
Abstract
Abstract: E928
Type: Eposter Presentation
Background
Personalized medicine will strengthen the prognosis and provide better, patient-specific drug identification. This type of clinical approach is based on high throughput analysis technology available today. Cancer could benefit from targeted therapy because of high number and heterogeneity of DNA alterations. Acute Myeloid Leukemia (AML) is characterized by several type of known point mutations and chromosomal aberration. Massive parallel sequencing showed that a number of new discovered single nucleotide variants (SNV) could affect patients survival time and drug resistance. In this clinical settings next generation sequencing (NGS) is becoming a useful tool to investigate patients genome giving a detailed picture of which mutations occurred and hence an improved diagnosis.
Aims
To exploit these informations for prognosis and personalized therapies there is the need of easily available tools for clinicians. We propose a statistical model learned from data provided by NGS technology, patients cytogenetics predictive risk and clinical outcomes, which could be useful to predict drug sensitivity and prognosis for new diagnosed patients.
Methods
DNA from a cohort of 65 AML patients from S. Orsola University Hospital, Bologna, Italy, were analyzed by Whole Exome Sequencing (WES). Different computational tools were assembled in an in-house pipeline in order to achieve an optimal detection lowering false positive rate. Briefly, pre-processing was carried out following GATK guidelines and re-calibrated alignment files were analyzed by MUTECT and VARSCAN2 algorithms in order to detect single nucleotide variants (SNV) and indels. An additional AML dataset containing WES data from 200 AML patients were downloaded from TCGA database in order to collect somatic variants and clinical data. A training set (60 percent of cases) was built stratifying patients by age and sex in order to maintain the proportion of the original entire dataset. Other 30 percent of cases were employed as testing set.SNV for each patients were mapped to Reactome and Pathways Commomns. Binary matrix factorization was applied to the patients/pathways matrix in order to find pathways signatures capable to cluster patients. To model pathways constrained mutational data toward event free survival or drug resistance we employed a generalized linear model with binary outcome, including also cytogenetics risk and presence/absence of cancer genes driver mutations from AML biomedical literature. Regression model was applied with 10-fold cross validation to find statistically significant explanatory variables.
Results
Whole exome sequencing detected 4783 somatic mutations of which 2441 labeled as non-silent. Non-silent mutations were mapped to pathways databases and binary matrix factorization. Five pathways signatures were obtained allowing clustering of patients in three groups. Recurrent driver genes involved in AML onset were found with different frequencies in patients and included in the model. Regression analysis provided a model which allow classification of patients in responder/non-responder to standard chemotherapy and toward event free survival.
Conclusion
In the complex task of interpreting NGS data collected from AML patients, this tool could be useful to guide clinician in the selection of a standard therapy versus experimental therapy: when poor prognosis or drug resistance is more likely associated with patients mutational data, latter could be preferable.Acknowledgements: ELN, AIL, AIRC, PRIN, Progetto Regione-Università 2010-12 (L. Bolondi), FP7 NGS-PTL project.
Session topic: E-poster
Keyword(s): Acute leukemia
Type: Eposter Presentation
Background
Personalized medicine will strengthen the prognosis and provide better, patient-specific drug identification. This type of clinical approach is based on high throughput analysis technology available today. Cancer could benefit from targeted therapy because of high number and heterogeneity of DNA alterations. Acute Myeloid Leukemia (AML) is characterized by several type of known point mutations and chromosomal aberration. Massive parallel sequencing showed that a number of new discovered single nucleotide variants (SNV) could affect patients survival time and drug resistance. In this clinical settings next generation sequencing (NGS) is becoming a useful tool to investigate patients genome giving a detailed picture of which mutations occurred and hence an improved diagnosis.
Aims
To exploit these informations for prognosis and personalized therapies there is the need of easily available tools for clinicians. We propose a statistical model learned from data provided by NGS technology, patients cytogenetics predictive risk and clinical outcomes, which could be useful to predict drug sensitivity and prognosis for new diagnosed patients.
Methods
DNA from a cohort of 65 AML patients from S. Orsola University Hospital, Bologna, Italy, were analyzed by Whole Exome Sequencing (WES). Different computational tools were assembled in an in-house pipeline in order to achieve an optimal detection lowering false positive rate. Briefly, pre-processing was carried out following GATK guidelines and re-calibrated alignment files were analyzed by MUTECT and VARSCAN2 algorithms in order to detect single nucleotide variants (SNV) and indels. An additional AML dataset containing WES data from 200 AML patients were downloaded from TCGA database in order to collect somatic variants and clinical data. A training set (60 percent of cases) was built stratifying patients by age and sex in order to maintain the proportion of the original entire dataset. Other 30 percent of cases were employed as testing set.SNV for each patients were mapped to Reactome and Pathways Commomns. Binary matrix factorization was applied to the patients/pathways matrix in order to find pathways signatures capable to cluster patients. To model pathways constrained mutational data toward event free survival or drug resistance we employed a generalized linear model with binary outcome, including also cytogenetics risk and presence/absence of cancer genes driver mutations from AML biomedical literature. Regression model was applied with 10-fold cross validation to find statistically significant explanatory variables.
Results
Whole exome sequencing detected 4783 somatic mutations of which 2441 labeled as non-silent. Non-silent mutations were mapped to pathways databases and binary matrix factorization. Five pathways signatures were obtained allowing clustering of patients in three groups. Recurrent driver genes involved in AML onset were found with different frequencies in patients and included in the model. Regression analysis provided a model which allow classification of patients in responder/non-responder to standard chemotherapy and toward event free survival.
Conclusion
In the complex task of interpreting NGS data collected from AML patients, this tool could be useful to guide clinician in the selection of a standard therapy versus experimental therapy: when poor prognosis or drug resistance is more likely associated with patients mutational data, latter could be preferable.Acknowledgements: ELN, AIL, AIRC, PRIN, Progetto Regione-Università 2010-12 (L. Bolondi), FP7 NGS-PTL project.
Session topic: E-poster
Keyword(s): Acute leukemia
Abstract: E928
Type: Eposter Presentation
Background
Personalized medicine will strengthen the prognosis and provide better, patient-specific drug identification. This type of clinical approach is based on high throughput analysis technology available today. Cancer could benefit from targeted therapy because of high number and heterogeneity of DNA alterations. Acute Myeloid Leukemia (AML) is characterized by several type of known point mutations and chromosomal aberration. Massive parallel sequencing showed that a number of new discovered single nucleotide variants (SNV) could affect patients survival time and drug resistance. In this clinical settings next generation sequencing (NGS) is becoming a useful tool to investigate patients genome giving a detailed picture of which mutations occurred and hence an improved diagnosis.
Aims
To exploit these informations for prognosis and personalized therapies there is the need of easily available tools for clinicians. We propose a statistical model learned from data provided by NGS technology, patients cytogenetics predictive risk and clinical outcomes, which could be useful to predict drug sensitivity and prognosis for new diagnosed patients.
Methods
DNA from a cohort of 65 AML patients from S. Orsola University Hospital, Bologna, Italy, were analyzed by Whole Exome Sequencing (WES). Different computational tools were assembled in an in-house pipeline in order to achieve an optimal detection lowering false positive rate. Briefly, pre-processing was carried out following GATK guidelines and re-calibrated alignment files were analyzed by MUTECT and VARSCAN2 algorithms in order to detect single nucleotide variants (SNV) and indels. An additional AML dataset containing WES data from 200 AML patients were downloaded from TCGA database in order to collect somatic variants and clinical data. A training set (60 percent of cases) was built stratifying patients by age and sex in order to maintain the proportion of the original entire dataset. Other 30 percent of cases were employed as testing set.SNV for each patients were mapped to Reactome and Pathways Commomns. Binary matrix factorization was applied to the patients/pathways matrix in order to find pathways signatures capable to cluster patients. To model pathways constrained mutational data toward event free survival or drug resistance we employed a generalized linear model with binary outcome, including also cytogenetics risk and presence/absence of cancer genes driver mutations from AML biomedical literature. Regression model was applied with 10-fold cross validation to find statistically significant explanatory variables.
Results
Whole exome sequencing detected 4783 somatic mutations of which 2441 labeled as non-silent. Non-silent mutations were mapped to pathways databases and binary matrix factorization. Five pathways signatures were obtained allowing clustering of patients in three groups. Recurrent driver genes involved in AML onset were found with different frequencies in patients and included in the model. Regression analysis provided a model which allow classification of patients in responder/non-responder to standard chemotherapy and toward event free survival.
Conclusion
In the complex task of interpreting NGS data collected from AML patients, this tool could be useful to guide clinician in the selection of a standard therapy versus experimental therapy: when poor prognosis or drug resistance is more likely associated with patients mutational data, latter could be preferable.Acknowledgements: ELN, AIL, AIRC, PRIN, Progetto Regione-Università 2010-12 (L. Bolondi), FP7 NGS-PTL project.
Session topic: E-poster
Keyword(s): Acute leukemia
Type: Eposter Presentation
Background
Personalized medicine will strengthen the prognosis and provide better, patient-specific drug identification. This type of clinical approach is based on high throughput analysis technology available today. Cancer could benefit from targeted therapy because of high number and heterogeneity of DNA alterations. Acute Myeloid Leukemia (AML) is characterized by several type of known point mutations and chromosomal aberration. Massive parallel sequencing showed that a number of new discovered single nucleotide variants (SNV) could affect patients survival time and drug resistance. In this clinical settings next generation sequencing (NGS) is becoming a useful tool to investigate patients genome giving a detailed picture of which mutations occurred and hence an improved diagnosis.
Aims
To exploit these informations for prognosis and personalized therapies there is the need of easily available tools for clinicians. We propose a statistical model learned from data provided by NGS technology, patients cytogenetics predictive risk and clinical outcomes, which could be useful to predict drug sensitivity and prognosis for new diagnosed patients.
Methods
DNA from a cohort of 65 AML patients from S. Orsola University Hospital, Bologna, Italy, were analyzed by Whole Exome Sequencing (WES). Different computational tools were assembled in an in-house pipeline in order to achieve an optimal detection lowering false positive rate. Briefly, pre-processing was carried out following GATK guidelines and re-calibrated alignment files were analyzed by MUTECT and VARSCAN2 algorithms in order to detect single nucleotide variants (SNV) and indels. An additional AML dataset containing WES data from 200 AML patients were downloaded from TCGA database in order to collect somatic variants and clinical data. A training set (60 percent of cases) was built stratifying patients by age and sex in order to maintain the proportion of the original entire dataset. Other 30 percent of cases were employed as testing set.SNV for each patients were mapped to Reactome and Pathways Commomns. Binary matrix factorization was applied to the patients/pathways matrix in order to find pathways signatures capable to cluster patients. To model pathways constrained mutational data toward event free survival or drug resistance we employed a generalized linear model with binary outcome, including also cytogenetics risk and presence/absence of cancer genes driver mutations from AML biomedical literature. Regression model was applied with 10-fold cross validation to find statistically significant explanatory variables.
Results
Whole exome sequencing detected 4783 somatic mutations of which 2441 labeled as non-silent. Non-silent mutations were mapped to pathways databases and binary matrix factorization. Five pathways signatures were obtained allowing clustering of patients in three groups. Recurrent driver genes involved in AML onset were found with different frequencies in patients and included in the model. Regression analysis provided a model which allow classification of patients in responder/non-responder to standard chemotherapy and toward event free survival.
Conclusion
In the complex task of interpreting NGS data collected from AML patients, this tool could be useful to guide clinician in the selection of a standard therapy versus experimental therapy: when poor prognosis or drug resistance is more likely associated with patients mutational data, latter could be preferable.Acknowledgements: ELN, AIL, AIRC, PRIN, Progetto Regione-Università 2010-12 (L. Bolondi), FP7 NGS-PTL project.
Session topic: E-poster
Keyword(s): Acute leukemia
{{ help_message }}
{{filter}}